MapR Converged Data Platform was MapR product. HPE acquired business assets in August 2019. MapR platform is now the integral part of HPE Ezmeral platform.
What can it offer and how can we use it? Software-defined storage has made a difference in how companies perceive and use data at their disposal. Distributed file systems enabled businesses to better manage their data – locally and in the cloud. Data environments became way easier to provision; business users can now equip the data with different rules and policies. They dictate if and when the data moves to a different storage tier directly inside the distributed file system, without external data movement applications.
Such environments are scalable, high performing, readily available, and reliable under all demanding circumstances without sacrificing security and environment simplicity. It also becomes even more attractive if they run on the so-called white box infrastructure; meaning they are not dependent on specialized appliances or hardware providers. And in the case of MapR there is no cloud or hardware lock-in. So what is MapR all about? “All” in this case actually means just about everything one can envision under the data umbrella. It includes file storage, streaming, NoSQL technologies or storing data for containerized applications.
Efficient data management will help you build applications and data services faster. What you probably don’t know is that you can do all of it under one roof. MapR Converged Data Platform is a highly integrated enterprise storage software. It brings software-defined storage, big data challenges, and data science environments to a whole another level. A platform for all the data and applications
A platform for all the data and applications.
The beauty of MapR lies in its carefully planned storage architecture. With MapR, users have a single platform (on a single codebase!) that delivers data-wide convergence. It is the only platform that has a distributed file system which supports storage and analytics of data streams, files and NoSQL tables in the same converged platform. This architecture also features a multi-model NoSQL database, event streaming engine, several querying tools with ANSI SQL support, and a broad set of open-source data management and analytics frameworks. There is support for technologies such as Hive, HBase, Spark, Drill and others which enable the development of robust and reliable data-oriented applications in a common, convergent environment.
The platform supports the creation of both batch and real-time applications using large data loads or small incremental events. Managing tons of data across different systems, applications and silos is generally complex and a true pain – but significantly less so when using
The MapR is providing speed, scale, and reliability for both operational and analytical workloads in a single platform. And the fact that it runs with great results on commodity hardware is another benefit. It just works with whatever you throw at it – even exabytes of data. And there is everything else that one would expect from an enterprise level data platform – it checks the right boxes when it comes to high availability (HA), disaster recovery (DR), data recovery and uptime guarantees. Furthermore, a unified security solution watches over all platform components at all time.
Unleash the power of big data
The big data environments had an association with the Hadoop ecosystem in the past, but MapR’s implementation goes past that. Spark, Hdfs, Kafka, and a host of other open-source technologies are tightly integrated and managed within a single platform. That proves that breaking down data silos is possible and shows the way it should be done. The platform’s unique architectural design reflects in the distributed filesystem MapR-XD which simply eliminates the limitations imposed by typical big data environments that require several separated siloes for addressing different kinds of workloads. On top of that, MapR is Posix compliant, supports random read/write operations and simplifies data movement with the support of NFS and data movement policies.
Spoil your data scientists
Once the company has all its data in order it is time to put it to work. In order to do so, the company has to create a data science environment and employ people who know to extract value from data. And since data scientists like spending their time on data-related business challenges and not tinkering with tools and data silos, they should get a reward – technology-wise. MapR takes a holistic approach to self-service data science. A preconfigured Docker environment in shape of a Data Science Refinery is equipped with the latest advanced analytics tools such as Drill, Spark, Hive and Zeppelin. These can be paired with several solutions such as TensorFlow, Caffe, MXNet, H2O and others.
Today, data scientists on average spend 80% of their time on cleaning and processing of data. If those tasks can be quicker, their job would be a lot easier and more productive – and the scientists could focus on pressing data-related business challenges.
Even simplified access to data (eg. NFS) can go a long way. There are several other benefits of building a data science environment with MapR. Data and job processing placement on different types of hardware is just one of them (eg. SSD vs HDD, CPU vs GPU).
Apps, containers and microservices
The architecture of the MapR Converged Data Platform is not just about big data. Its data-centric vision also takes into account the new generation of applications – and even those that we are yet to build tomorrow. Modern applications rely on microservices to get the right data in the right way for them to perform business tasks. We can view microservices themselves as single-purpose applications or funciton that work in unison via lightweight communications, such as data streams and deliver a simplified way to build and integrate modern applications in ways that have traditionally been impossible with monolithic applications. MapR’s platform supports building event-driven microservices that leverage event streaming technologies (like MapR-ES) as the communications vehicles. By converging file, database, and streaming services one can develop agile applications for various types of workloads and business needs – for example: real-time, batch or advanced analytics applications.
But storing data for microservices, especially those running in containers, have proven to be quite challenging. Especially for traditional storage technologies due to lack of connectivity to the container ecosystem and inability to meet performance and scaling requirements. Despite the stateless and ephemeral nature of containers in most cases, their data still have to live somewhere. This can be a distributed file system, NoSQL database, a streaming solution or other dedicated storage technology. MapR provides an ideal platform for such cases and can leverage all of its platform storage services as a long-living persistent data store.
Cutting backup, archiving, and retention costs
We usually associate backups, compliance archiving and other secondary data with high storage costs and tedious retention processes. MapR’s platform takes into account data tiering and can automatically transfer older data to dense-storage and other cost-optimized locations (eg Amazon S3). Its cluster topology devoted to data archiving is known for high capacity/density and modest with system resources. In any case, the cost-effective data storage is aimed at ensuring regulatory compliance and different legal requirements.
Wrapping up
MapR Converged Data Platform was not the first on the market. However, it is the first one to deliver a unified and centralized management of data in a common platform. That’s archived by combining different types and forms of data without the use of separate specialized technologies. Its distributed file system handles files, documents, data streams, and tables under one roof. It facilitates the data storage, and maintenance, thus easing the administration burden. It does so in a cost-effective way. And it’s not picky. It simply handles all the data on-premise, in the cloud or at the edge of the network; and can scale-out to practically any workload.
Previous & Next
Building a Big Data Platform
Architecting, designing and building a robust and reliable analytics platform is an intricate process. Building a big data platform requires careful analysis an...
read more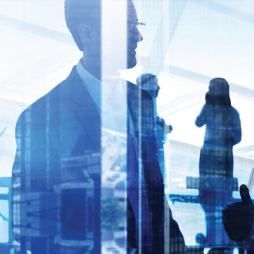
Need a Great Data Management Recipe? Look no Further.
If you need need a great data management recipe, you will find here. Data management is a complex field that is becoming even more complex. There are several fa...
read more